The problem
Currently, the aviation industry is losing more than half a billion dollars each year and has had more than 350 casualties in the last century. The culprit for this is not extreme storms, but surprisingly wildlife on the landing track and in the air space. As a result, the aviation industry invested in making planes more durable to counter animal strikes and instructed pilots to wait until it was safe to land on the landing track if an animal was present.
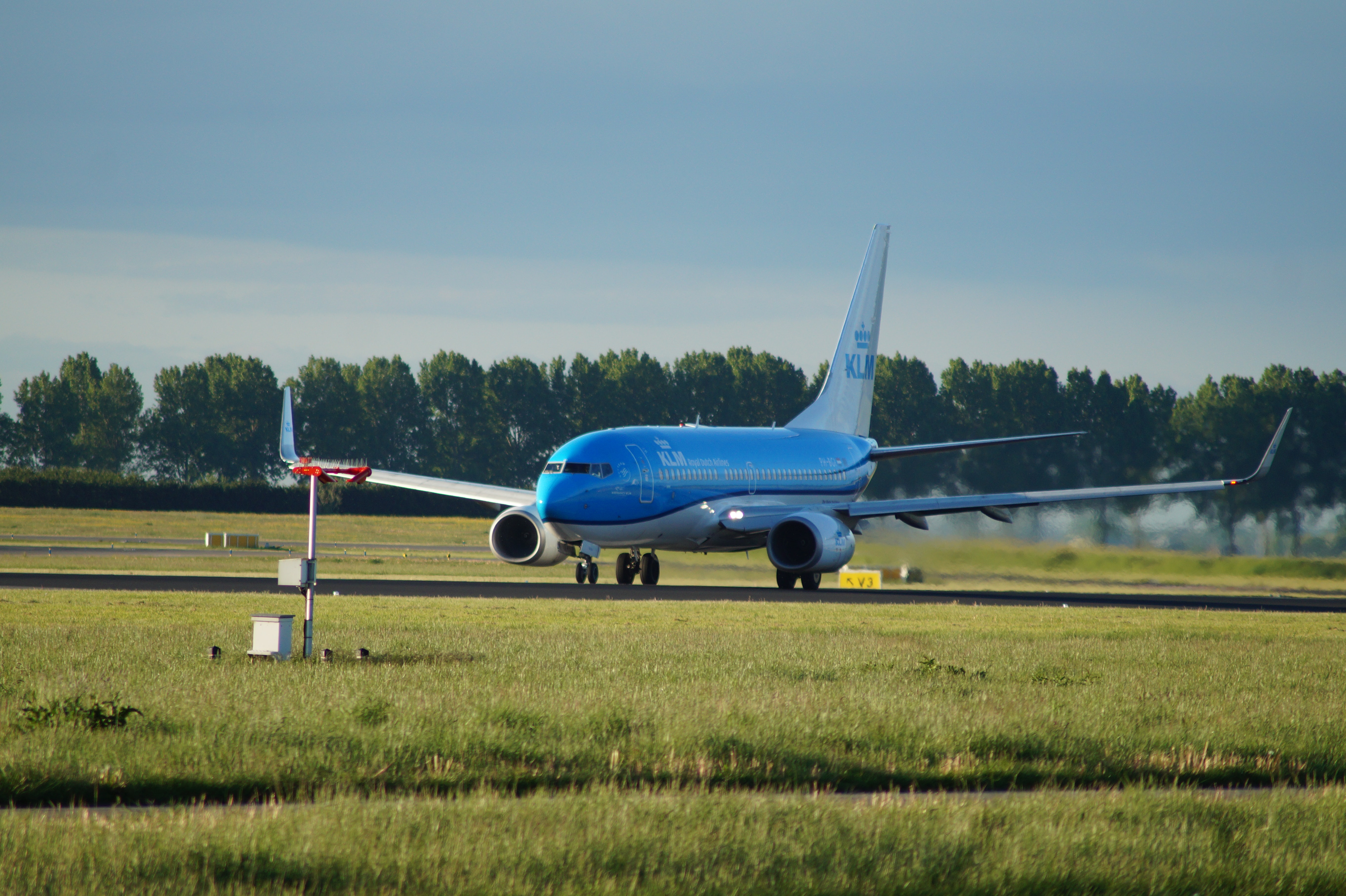
The solution
The above solutions are reasonable to a certain point, but there is still room for improvement to prevent animal collisions with an aircraft. One such solution is using A.I. to predict the animal's path before the plane lands on the runway.
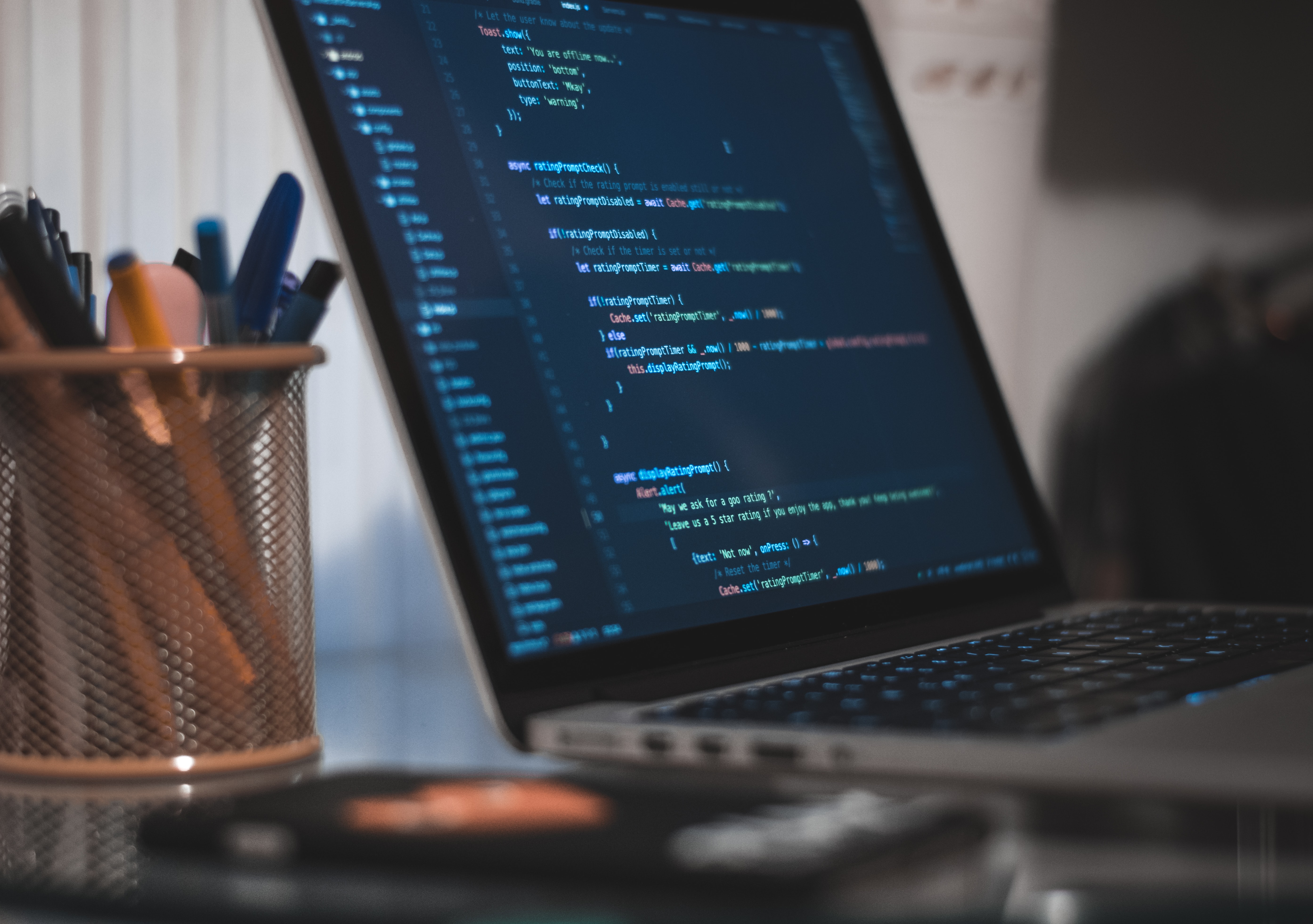
About AWIR
Creating an Aerial Wildlife Imagery Repository from Small Unmanned Aircraft Systems for Training Automated Image Classifiers (Original Proposal-2021)
Meilun Zhou and Dr. Sathish Samiappan, Geosystems Research Institute, Mississippi State University
Dr. Raymond Iglay1, Dr. Kristine Evans, and Dr. Jared Elmore, Department of Wildlife, Fisheries, and Aquaculture, Mississippi State University
The increased use of small (<55lbs) unmanned aircraft systems (sUAS) to survey wildlife presents a need and opportunity for researchers to train computer vision algorithms on sUAS imagery collected to assist with animal detection. Specifically, a continuously refined computer vision “system” of algorithms will provide federal agencies and public entities interested in wildlife surveys via sUAS enhanced ability to detect and accurately count wildlife. We see this capability as important to understanding negative human-wildlife interactions and conservation. As such, we propose an Aerial Wildlife Image Repository (AWIR), a suite of opensource datasets which will be annotated from both aerial images and videos (hereafter referred to as data) captured from sUAS. These data will be available to train machine learning and computer vision algorithms that can detect wildlife from aerial imagery. Currently no repository exists publicly for this purpose, and AWIR would be the first of its kind.
Computer vision algorithms for recognizing objects in images learn through large amounts of data (supervised learning). Therefore, a primary purpose of AWIR will be improving computer recognition of wildlife in images captured from sUAS. The existence of datasets drives machine learning. One example of a successful dataset is CIFAR-10, a benchmark dataset used to train several machine learning networks, consists of 60,000 color images split among 10 classes. The existence of this dataset has proved extremely beneficial to training classifiers by providing a benchmark for researchers to test their algorithms and compare methods. The availability of an ever-improving repository of datasets allows machine learning algorithms to have a robust training set of images, which in turn allows for accurate detection and classification of wildlife. Some individual datasets are publicly available but having a well-organized collection reduces the time needed to search for individual animal datasets.
In order to train machine learning models for wildlife, each dataset will need images with and without animals. Also, an ideal dataset should contain multiple postures of each species (e.g., standing, lying down, flying, perched, etc.). Images of occluded animals will also be considered for inclusion in the dataset. A preliminary target of at least 80 images per animal class or species should suffice. Once a dataset contains the preliminary target of 80 images, it is considered complete and will be made publicly available on AWIR. With widescale use, the compiled datasets of animals would allow researchers access to a large variety of animal imagery to train different algorithms. There will be N number of datasets within AWIR, which will contain visible and thermal infrared images. N will represent different classes of identified mammalian and avian wildlife. Focal species and faunal groups for AWIR datasets will be decided based on species and image availability, with a proposed sampling priority given to wildlife species hazardous to aircraft, common wildlife damage-associated species, and other common wildlife species. Subsequent datasets could be populated with less common wildlife species or species harder to monitor with sUAS (i.e., highly mobile species).
The AWIR will be accompanied with a classification benchmarking website showcasing examples of state-of-the-art algorithms recognizing the wildlife in the images. For many academic and industry researchers, the availability of a truth-labeled test dataset helps drive algorithm research, and AWIR will be a unique repository of datasets for wildlife detection/classification from sUAS-collected imagery. With increased use, AWIR can be used to house imagery of non-terrestrial wildlife and other animal taxa. The organizations involved in developing AWIR will be acknowledged in the form of publication citations from those who used AWIR in their studies. This is the norm when it comes to dataset acknowledgment.
The AWIR database will be developed by the Mississippi State University (MSU) team in collaboration with USDA Wildlife Services (USDA WS), and the Federal Aviation Administration (FAA). All three entities will play integral roles in developing the functionality of AWIR considering image submission, image QA/QC, maintenance of the complete image library (all images), prioritizing species and research needs, coordinating interests among multiple stakeholders, evaluating the repositories before their release, advertising AWIR, and managing the functionality of the repository.